Legionella Exposure Risk in Groundwater Treatment Plants
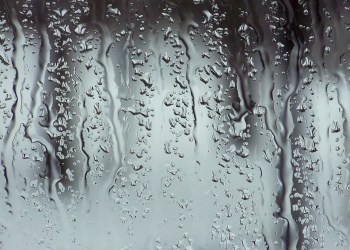
Using Bayesian models to assess exposure risks for aeration systems.
DOWNLOAD THE PAPER
Abstract
Aeration systems used in groundwater treatment plants are perceived as a potential source of Legionella exposure, an opportunistic pathogen of significant public health concern. Building on the Legionella High Level Risk Assessment Initiative (LHLRAI) developed by Water Corporation Western Australia, this study aims to further quantify the potential of Legionella exposure. Characterisation of fouling samples from seven aerators concluded that iron was the most abundant element in the foulant (up to 80%) which promotes Legionella growth.
Guided by the LHLRAI, sampling analysis, and site observations, Legionella exposure risk was modelled using Bayesian Networks (BN). The input variables – nutrient availability, stagnant water, system deficiencies, and location and access, as well as their associated weighting scores – were represented in the BN model. The output states of Legionella exposure were uniformly discretised into three states: low, medium, and high. A low state requires no mitigative action; the medium state prompts the review of operational parameters and high state calls for immediate decontamination. The model predicted Legionella exposure risk for tray and spray aerators to be medium and low respectively. Subsequent sensitivity analysis indicated that poor water quality presented the strongest influence on Legionella exposure followed by location and access to the system for maintenance. This initial assessment demonstrates the potential of BN modelling to improve risk assessments that support practical decision-making in managing Legionella exposure.
Introduction
Legionella occurs naturally in freshwater environments (at low concentration) but grows in a wide variety of engineered water systems that provide favourable conditions (van Heijnsbergen et al., 2015). Human exposure is via the inhalation of aerosols contaminated with the bacteria (Fraser, 1980). Outbreaks of Legionnaires’ disease have been tracked in engineering water systems such as plumbing systems (e.g. showers, faucets, and toilets), cooling towers, respiratory devices (e.g. humidifiers, vaporisers, and nebulisers), swimming pools (including spas/hot tubs and whirlpools), steam-producing appliances, and ornamental fountains) (van Heijnsbergen et al., 2015).
Despite, the significant improvement in our understanding of Legionella ecology including the emergence of standards and guidelines (aimed at preventing their multiplication in risky systems) (AS/NZS 3666, 2011; AS 5059, 2006), managing their exposure remains a critical challenge. Legionnaires’ disease outbreak is on the rise globally with cooling towers being the common source of transmission (Walser et al., 2014). Studies indicated many other potential sources of Legionella exposure have been overlooked (Coleman, 1998; Prussin et. al, 2017).
Engineered aeration systems commonly used in drinking water plants are perceived as one of such overlooked Legionella transmission sources. Aeration units are employed to treat groundwater for potable uses. In Western Australia, tray and spray aeration systems are commonly employed as a pre-treatment process in drinking water plants (Figure 1).
Until now, no Legionella outbreak has been linked to the treatment plants, largely due to the multiple and robust treatment barriers in place.
Figure 1. (a) Spray aeration and (b) Multi-tray aerator units in groundwater treatment plants in Western Australia
However, there is a growing concern that fouling deposits after prolonged aeration operation can provide favourable conditions for Legionella growth, which is exacerbated by its preferred growth in warm water (37 – 42°C). Thus, Water Corporation Western Australia (WCWA) deems these assets to pose an unacceptable Legionella risk to the health of operators and the local community.
The potential of Legionella growth in aerators is expected to be significantly under-recognised across the water sector and likely affects most drinking water treatment plants in hot climate regions worldwide.
Aerators have been shown to provide optimal growth conditions for aquatic bacteria with an aerobic metabolism, an example being the detection of Burkholderia in a spray aeration unit within a water treatment plant in Northern Australia (Inglis et al., 2000). Water quality parameters frequently associated with poor microbial quality such as organic carbon and turbidity as well as certain metals such as iron and manganese have shown positive effects on Legionella growth (Bargellini et al., 2011). Also, specific water system deficiencies, such as insufficient disinfection and improper maintenance have been implicated in numerous outbreaks across the world (Atlas, 1999; Walser et al., 2014). The possibility of similar events occurring in aeration units and facilitating rapid microbial population “blooms” resulting in transmission and subsequent increased risk of Legionellosis is not unrealistic considering that aerators are widely used as a pre-treatment option by water utilities (Munter et. al, 2005).
To ensure that aeration systems continue to serve their purpose of treating potable groundwater supplies in many communities in Australia, without contributing to the health burden of the public, WCWA initiated a Legionella High Level Risk Assessment Initiative (LHLRAI) to determine the risk level present in these assets. The assessment recognises that the current set of standards available for microbial management in air handling and water systems (AS/NZS 3666, 2011; AS 5059, 2006) is not fully appropriate for acceptable risk management of aerator systems.
Developed through expert elicitation, LHLRAI is a risk matrix that facilitates the inspection and assessment of aeration assets to ensure compliance with regulated conditions prescribed in the AS 3666, more specifically examining the presence of stagnant water, nutrient availability and growth, poor water quality, deficiencies in the system, as well as location and access points of the asset.
As a result, this study in collaboration with WCWA, builds on the LHLRAI to further quantify the potential for Legionella exposure in aeration assets through a range of risk management activities. A comprehensive inspection of existing aerator assets in Groundwater Treatment Plants (GWTPs) in Western Australia, covering the mid-west and south-west Perth regions, was completed to assess the potential risk. Targeted sampling and advanced characterisation of water and fouling deposits were conducted to systematically investigate and provide a better understanding of the parameters that promote and contribute to Legionella growth. The potential for Legionella growth leading to exposure through aerosolisation was characterised using a proposed predictive Bayesian Network (BN) model.
Site inspection and analysis
The aeration assets were inspected to identify potential events that may lead to Legionella exposure. Legionella growth in the aerator system is the ‘source’ with aerosol generation acting as the transmission ‘pathways’ to reach potential ‘receptors’ (plant operators and nearby communities). The presence of a source-receptor-pathway establishes the potential Legionella exposure risk.
The configuration of the aerators falls into two categories: tray and spray aeration systems (Figure 1), with intermittent operation in the regional plants and continuous operation in the metro spray aerator depending on the demand.
Due to the variations in the feed water quality, the age of the asset, and material composition, a wide range of aerator conditions were observed resulting in the maintenance and sampling from aeration systems fraught with challenges. Typically, the aerators were not adequately designed to allow convenient isolation from downstream equipment (filters and clearwater tanks) in order to enable efficient and safe in-situ flushing and cleaning. Particularly, tray aeration systems required cutting out the grills to allow for sample collection and maintenance access.
Like other dynamic water systems, biofouling slime, iron precipitates, and biofilms in the aeration sump were clearly visible (Figure 2) despite the routine cleaning and maintenance conducted by the water utility. The observed slime slurries and solid deposits were sampled from different locations within the spray and tray aeration systems for characterisation.
Figure 2. Spray aeration system with slime deposits and reddish-brown deposit from tray aeration units resulting in fouling
Foulants (deposits) characterisation
To determine the constituents of the deposits, gravimetric analysis described the moisture content (MC), total solids concentration (TS), and volatile organic solids. The elemental compositions were quantitatively analysed using inductively coupled plasma optical emission spectroscopy (ICP-OES), while the formation, composition, and structural patterns of the deposits were assessed via scanning electron microscope (SEM) and x-ray powder diffraction (XRD) analytical techniques. The elemental composition of the top five (5) most abundant elements is presented in Table 1.
For the GWTPs studied, the common matrix elements were iron, silicon, calcium, phosphorus, sodium, potassium, sulfur, barium, and magnesium. Iron was the most prevalent element constituent in most of the samples tested. The prevalence of iron in the elemental composition is considered predictable as aerators are designed to oxidise and precipitate iron from groundwater, which also explains the commonly observed phenomenon of reddish-brown staining of internal and external aerator surfaces.
The other common elements, in decreasing order of prevalence, were Si, P, Na, K, S, Ba, and Mg. The abundant concentration of iron in the deposit is likely to significantly influence habitat and nutrient conditions which support Legionella growth while Phosphorus, the fourth most abundant element found in the deposits sample, can be associated with the uptake of biofilm and cellular materials. The residual ash proportion after ignition at 550°C in the muffled furnace is representative of the total amount of inorganic substances within a sample. The ash proportion for most of the aerator samples was above 80%, indicating minimal levels of organic substances and further suggesting that most of the fouling samples were inorganic precipitates.
It is obvious that deposits within aerators at GWTPs may retain inorganic contaminants that promote the growth of Legionella with iron having been implicated to be a source of nutrient for Legionella (Portier et. al., 2016)
Table 1. Characteristics of slurry and solid deposits sampled from aerator systems
The SEM images (Figure 3) of the deposits show a uniform fouling distribution and the presence of fouling diatoms attributed to the development of biofilms (Molino, et.al, 2009). The XRD graphs (Figure 4) showed that calcite (CaCO3,), silicate (SiO2), and iron (Fe) were the major phases present.
This observation is consistent with the findings of Peng et al. and suggests that precipitation of calcite was from hard water (Peng et al., 2010). XRD characterisation of the samples used in this study provided an important insight into the nature of the prevalent solid phases formed in deposits from aeration systems.
To better assess the risk factor of iron precipitation at a site, further examination of the correlation between the levels of iron in the feedwater and the net amount of precipitation is required. Presence of a significant relationship would suggest that feedwater analysis can be used to predict aerator fouling propensity. The abundance of iron shows potential for Legionella growth in aerators, which is exacerbated by the uneven precipitation of calcite and silicate, will promote water stagnation. It is expected that deposition rates are inextricably linked to aerator design, which has not been traditionally considered in effective management of fouling and maintenance regimes.
Figure 3. Micrograph of the sampled deposit showing uniform formation and fouling diatoms
Figure 4. Primary crystalline phases from the sampled deposits
Exposure risk characterisation
The LHLRAI (as described in section 1) has provided some insights to understanding and quantifying Legionella exposure. However, the approach is limited by its inability to categorise the risk of different exposure levels and account for uncertainties in its predictions. As a result, a predictive BN model that incorporated the data collected during the site visits was introduced.
BNs are robust visualisation models that integrate probabilistic reasoning with graphical representation to successfully capture explicit causality relationships and different elements of uncertainty with a probabilistic outcome. BN modelling has shown great promise and is becoming an increasingly popular method for monitoring complex environmental systems (Barton et al., 2012; Carvajal et al., 2017; Beuzen et. al, 2018). BNs are also capable of developing predictive indicators to replace routine microbial monitoring of Giardia lamblia and Cryptosporidium parvum (Carvajal et al., 2015).
In this study, we present the initial development stage of the proposed BN model. The aim of the model is to improve understanding of the system parameters as well as identify knowledge and data gaps related to Legionella exposure in aeration systems. Guided by the LHLRAI as well as observations and findings from the site visits, the variables and their associated weighting scores were encoded and represented in the BN. The rationale and description of the variables in the model are summarised in Table 2.
Table 2. Rationale and description of model nodes
The input variables are nutrient availability, stagnant water, system deficiencies, and location and access. The output parameter ‘Legionella exposure’ represents the level of risk that was, so far, inadequately established within the LHLRAI. For the reason explained earlier, the BN offers a more accurate estimation of the level of that risk.
The weighted score for each of the variables was discretised into three states using a uniform distribution as summarised in Table 3. The BN model was built using the Netica software (Norsys Software Corp.) and calibrated with water quality and operational parameters obtained from the site visits and the LHLRAI.
Table 3. Description of states in the model nodes and their implications
Figure 5 shows the output BN model. The variables are graphically displayed with arcs depicting the causality relationships. Each state of the variable showed a probability distribution indicating the chance of occurrence of that particular state.
Figure 5. Bayesian network of Legionella exposure, with the five input nodes as defined in Table 2
The model output and interaction between variables are clearly displayed in a transparent manner compared to traditional black-box models. This promotes system learning and allows users to interrogate the reasoning behind the model outputs (Chen and Pollino, 2012). However, the defensibility of the interactions between the variables and the outcomes still need to be evaluated.
Ideally, the accuracy of the model is to be tested with empirical data. While the actual concentration of Legionella in the asset were used to populate node 5 (poor water quality), there was no existing empirical data to quantify the Legionella exposure node (due to the lack of approximate measuring strategies used so far). As a result, it was not possible to fully test the accuracy of the model.
To address this limitation, initial assessment of the model using case scenarios and sensitivity analysis are generally recommended (Aguilera et. al, 2011).
The model (Figure 5) was evaluated using two scenarios developed by assessing a set of input variables as summarised in Table 4. Field observation of parameters contributing to each of the model variable were assessed, and their corresponding weighed scores applied to derive states for the nodes. Applying the different inputs variables, the probabilities in the network were examined. The interaction of the variables and subsequent outcome is expected to provide insights into the consistency of the model’s predictions with current understanding about the system.
Table 4. Two different scenarios developed from the field inspection of the aeration systems
Through belief propagation using Bayes’ Theorem, the model absorbed the input scenarios, facilitated interactions, and produced diagnoses for the Legionella exposure nodes of spray and tray aeration assets as shown in Figure 6 and Figure 7 respectively. The state in the variable with the highest probability is considered the model prediction.
Figure 6. Scenario assessment of a field inference from a Spray aeration site
Figure 7. Scenario assessment of a field inference from a Tray aeration site
The model predicted the risk of Legionella exposure to be medium and low for the two scenarios, which reflected the ability of the model to update the output prediction based on the changes and interactions between the input variables. The difference between the spray and tray aerator scenarios are in their nutrient availability and stagnant water states. The predicted outputs support the current understanding that changes in variables including nutrient availability and stagnant water are critical determinants to Legionella exposure (van der Lugt et al., 2019). However, to better understand how the variables influence the outcome, a sensitivity analysis was conducted.
Sensitivity analysis identified the most important variables of the model and was used to calculate the sensitivity of the Legionella exposure node to input variables in all other nodes of the network. The result indicated that poor water quality presented the strongest influence followed by location and access to the system. The BN was built as a direct translation of the LHLRAI, and as such, the sensitivity analysis results reflected its definition and connectivity between the variables. This further supports the model consistency with existing knowledge (Schoen and Ashbolt, 2011; Prussin et. al, 2017) despite the present BN model containing some level of uncertainty.
The uncertainty is due to the limitations in current understanding of Legionella exposure and the lack of occurrence data to evaluate its performance accuracy. Therefore, it should be noted that the BN model is part of an ongoing iterative model development process which will be improved as more knowledge and data about the Legionella exposure becomes available.
The applicability of BN models to capture current knowledge of variables and Legionella exposure shows great potential to be developed into a decision-making support system to promote a more consistent risk assessment for aeration assets. The findings from the model will also advise water utilities on how to optimise cleaning and maintenance regimes to reduce the potential for Legionella growth and its subsequent exposure.
Conclusion
The presence of nutrients and location and access for maintenance in aeration assets used for groundwater treatment could promote the growth and transmission of Legionella, further confirmed these assets as potential exposure sources. The subsequent risk of exposure from these sources can be quantified with a BN model that combined all the causal variables. The model was evaluated using scenarios assessment and sensitivity analysis, however, there is a lack of data to evaluate the performance accuracy.
This initial assessment demonstrates the potential of BN modelling to improve risk assessments that support practical decision-making in managing Legionella exposure. However, it is important that water utilities collect more data through the incorporation of routine Legionella quantification in their water safety plans. These datasets will help improve predictive robustness and allow for subsequent refinement of the model. An approach to refining the model could be to delineate the exposure risk model into two distinct sub-models of growth and transmission as well as incorporating Legionella occurrence data to improve the accuracy of prediction.
About the authors
Dunladi Yunana | Danladi Yunana is a Scientia PhD scholar at the School of Chemical Engineering, UNSW Sydney. He received his Masters’ degree in Environmental Engineering from Imperial College London as a Commonwealth Scholar funded by the UK government.
Amos Branch | Dr. Amos Branch is a research associate at the Water Research Centre, in the School of Civil and Environmental Engineering, UNSW Sydney. Amos’ current research areas include management of health risks in water reycling, investigating transformation pharmaceuticals in biological treatment, validation of ultraviolet advanced oxidation processes and improving operability of membrane systems. Amos also acts as the NSW representative on the AWA National Young Water Professional Taskforce.
Keng Han Tng | Dr. Keng Han Tng is a Postdoctoral Research Associate at the School of Chemical Engineering, UNSW Sydney. Han’s recent research areas include reliability and optimisation of
production processes as well as design of waste management and recycling solutions for the various industries.
Ian Bradley | Ian is a Principle Asset Capability Manager for the Water Corporation of Western Australia. He has 33 years industry experience involving paper manufacturing and packaging, food manufacturing and packaging, and water utility industries covering reliability engineering, tactical and strategic asset and maintenance management, operations, process optimisation, people management, engineering planning and project development and execution. Ian is an accredited Engineer with the Engineering Council UK and an Affiliate of the Australian Asset Management Council of Australia.
Luke Zappia | Luke Zappia is a senior water treatment advisor for the Water Corporation of Western Australia. Luke has more than 25 years of industry and research experience in various roles in the water sector. He is a leading water quality scientist recognised at national and international levels for his contributions to improving industrial and environmental outcomes for the mining, water and bioremediation industries. He has published and co-authored over 35 papers in international scientific journals and over 300 technical reports to industry, regulatory agencies and community groups.
Pierre Le-Clech | Pierre Le-Clech is an Associate Professor at the School of Chemical Engineering at UNSW, Australia. He has researched many aspects of the water and wastewater treatments by membrane processes in particular. Pierre has recently led a milestone project, developing new set of national validation guidelines for MBR and RO used in water recycling.
References
Aguilera, P. A. et al. (2011) ‘Bayesian networks in environmental modelling’, Environmental Modelling & Software. Elsevier, 26(12), pp. 1376–1388. doi: 10.1016/J.ENVSOFT.2011.06.004.
Atlas, R. M. (1999) ‘Legionella: from environmental habitats to disease pathology, detection and control’, Environmental Microbiology, 1(4), pp. 283–293. doi: 10.1046/j.1462-2920.1999.00046.x.
Australian / New Zealand Standard TM Air-handling and water systems of buildings — Microbial control Part 1 : Design , installation and commissioning (2011) Water.
Bargellini, A. et al. (2011) ‘Parameters predictive of Legionella contamination in hot water systems: Association with trace elements and heterotrophic plate counts’, Water Research. Elsevier Ltd, 45(6), pp. 2315–2321. doi: 10.1016/j.watres.2011.01.009.
Barton, D. N. et al. (2012) ‘Bayesian networks in environmental and resource management’, Integrated Environmental Assessment and Management, 8(3), pp. 418–429. doi: 10.1002/ieam.1327.
Beuzen, T., Marshall, L. and Splinter, K. D. (2018) ‘A comparison of methods for discretizing continuous variables in Bayesian Networks’, Environmental Modelling & Software. Elsevier, 108, pp. 61–66. Doi: 10.1016/J.ENVSOFT.2018.07.007.
Carvajal, G. et al. (2015) ‘Modelling pathogen log10 reduction values achieved by activated sludge treatment using naïve and semi naïve Bayes network models’, Water Research. Pergamon, 85, pp. 304–315. doi: 10.1016/J.WATRES.2015.08.035.
Carvajal, G. et al. (2017) ‘Bayesian belief network modelling of chlorine disinfection for human pathogenic viruses in municipal wastewater’, Water Research. Pergamon, 109, pp. 144–154. doi: 10.1016/J.WATRES.2016.11.008.
Chen, S. H. and Pollino, C. A. (2012) ‘Good practice in Bayesian network modelling’, Environmental Modelling & Software, 37, pp. 134–145. doi: 10.1016/j.envsoft.2012.03.012.
Coleman, J. (1998) ‘Considerations to Prevent Growth and Spread of Legionella in HVAC Systems.’, American School and Hospital Maintenance. Available at: https://eric.ed.gov/?id=EJ566348 (Accessed: 13 April 2019).
Fraser, D. W. (no date) Legionellosis: evidence of airborne transmission. Available at: nyaspubs.onlinelibrary.wiley.com/doi/pdf/10.1111/j.1749-6632.1980.tb18906.x (Accessed: 10 May 2019).
van Heijnsbergen, E. et al. (2015) ‘Confirmed and Potential Sources of Legionella Reviewed’, Environmental Science & Technology. American Chemical Society, 49(8), pp. 4797–4815. doi: 10.1021/acs.est.5b00142.
Inglis, T. J. et al. (2000) ‘Burkholderia pseudomallei traced to water treatment plant in Australia.’, Emerging infectious diseases. Centers for Disease Control and Prevention, 6(1), pp. 56–9. doi: 10.3201/eid0601.000110.
van der Lugt, W. et al. (2019) ‘Wide-scale study of 206 buildings in the Netherlands from 2011 to 2015 to determine the effect of drinking water management plans on the presence of Legionella spp.’, Water Research. Pergamon, 161, pp. 581–589. doi: 10.1016/J.WATRES.2019.06.043.
Marcot, B. G. et al. (2007) ‘Guidelines for developing and updating Bayesian belief networks applied to ecological modeling and conservation’, Canadian Journal of Forest Research, 36(12), pp. 3063–3074. doi: 10.1139/x06-135.
Molino, P. J., Campbell, E. and Wetherbee, R. (2009) ‘Development of the initial diatom microfouling layer on antifouling and fouling-release surfaces in temperate and tropical Australia’, Biofouling. Taylor & Francis , 25(8), pp. 685–694. doi: 10.1080/08927010903089912.
Munter, R., Ojaste, H. and Sutt, J. (2005) ‘Complexed Iron Removal from Groundwater’, Journal of Environmental Engineering, 131(7), pp. 1014–1020. doi: 10.1061/(asce)0733-9372(2005)131:7(1014).
Peng, C.-Y. et al. (2010) ‘Characterization of elemental and structural composition of corrosion scales and deposits formed in drinking water distribution systems’, Water Research. Pergamon, 44(15), pp. 4570–4580. doi: 10.1016/J.WATRES.2010.05.043.
Portier, E. et al. (no date) ‘Iron Availability modulates the persistence of Legionella pneumophila in complex biofilms’, jstage.jst.go.jp. Available at: www.jstage.jst.go.jp/article/jsme2/advpub/0/advpub_ME16010/_article/-char/ja/ (Accessed: 10 May 2019).
Prussin, A. J., Schwake, D. O. and Marr, L. C. (2017) ‘Ten questions concerning the aerosolization and transmission of Legionella in the built environment’, Building and Environment. Elsevier Ltd, 123, pp. 684– 695. doi: 10.1016/j.buildenv.2017.06.024.
Schoen, M. E. and Ashbolt, N. J. (2011) ‘An in-premise model for Legionella exposure during showering events’, Water Research. Pergamon, 45(18), pp. 5826–5836. doi: 10.1016/J.WATRES.2011.08.031.
Standards Australia (2006) ‘Power station cooling tower water systems—Management of legionnaires’ disease health risk’. Available at: www.standards.com.au
Walser, S. M. et al. (2014) ‘Assessing the environmental health relevance of cooling towers – A systematic review of legionellosis outbreaks’, International Journal of Hygiene and Environmental Health. Urban & Fischer, 217(2–3), pp. 145–154. doi: 10.1016/J.IJHEH.2013.08.002.