Enhancing Water Meter In-Service Testing and Replacement Decisions
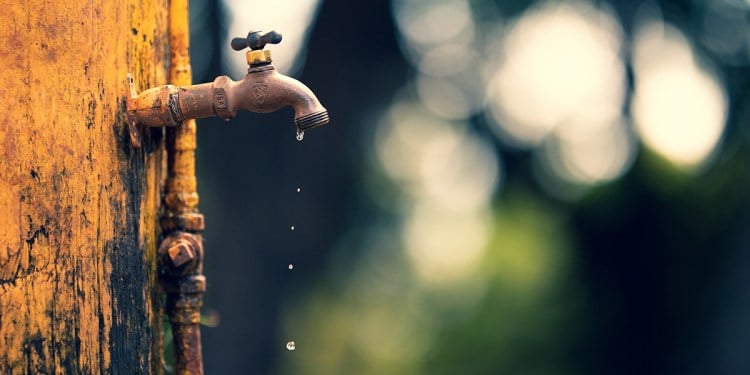
Achieving sustainable benefits for the community.
DOWNLOAD THE PAPER
Abstract
Currently water meter in-service testing and replacement decisions are guided by existing Australian regulations, standards and guidelines. There remains a need for additional methods/techniques to enhance this decision-making process and to quantify the volume of apparent water losses. In addition to meeting these needs, these additional methods/techniques should also facilitate attaining sustainable benefits for all metered customers and related stakeholders due to improvements in the accuracies of in-service meters.
Current generalised default values for average demands and usage profile weightings are not necessarily appropriate for all mechanical meter applications. Testing the statistical significance of error decay models based on fitting a linear regression curve to the results of six-flow rate point test results provides a more accurate and objective approach to assessing the relative weighted error and volume of apparent water losses.
Optimal replacement theory that considers the time value of money provides a useful technique to identify the impact that error decay rates have on meter replacement periods. Indications are that physical water quality characteristics such as high water temperatures and level of free chlorine accelerate wear and tear of mechanical meters. Comparison of a meter’s flow range capabilities with that of a customer’s historic water billing records facilitates identifying those cohorts potentially subjected to an increased rate of mechanical wear and tear.
Introduction
Supervising commercial practices to ensure honesty and integrity during the exchange of fixed amounts or measures of a product for money is not new. This was evident several thousand years ago when ancient scribes expressed their concerns about faulty measuring scales, which the civil authorities appeared to not adequately supervise (NIV Bible, 1984). However, sometimes the accuracy of a measuring device could unintentionally be adversely affected by human error resulting in a person omitting to do something, doing the wrong thing or undertaking something out of sequence (Johnson, 2009).
The modern science of measurement has a theoretical and experimental basis for the determination of accuracy or more correctly stated, the level of uncertainty of a measurement. This is especially relevant because no measurement is free from error and must be reported as a range and not as a single value. National and international metrological legislation and regulations set out the conditions with which a measuring device must comply in order to meet their requirements when they are subject to State controls. The procedures, methods and processes that an organisation must comply with within the context of these State controls is detailed in an applicable Quality System that facilitates the confidence of stakeholders in the measuring results produced by an organisation.
Decisions regarding water meter in-service testing and replacements are guided by current Australian standards and codes. However, these national standards and codes do not necessarily provide all the answers required to assess the in-service condition of the meter fleet so as to facilitate optimal decisions regarding their testing and replacements of which, the following are examples:
- Establishing the accuracy of current default values and the impact that these default values have on the results produced. An example of this is that in order to convert a meter’s flow accuracy test results into a combined weighted volumetric error, weightings for each flow rate range based on a typical water usage/consumption pattern is applied. Australian Standard AS 3565.4 (2007) provides default weightings that do not necessarily apply to the ‘average’ customer across Australia.
- Correct interpretation of the results from test samples taken from a population as well as using these results to estimate the volume of apparent water losses. Applicable in-service standard (AS 3565.4) and code (WSA 11) do not detail how the volume of water loss can be estimated for the whole meter fleet.
- Assessing the potential impact that changes in metering technologies have on current regulations and standards that are based on the operational characteristics of mechanical meters originally designed over 100 years ago. These commonly adopted positive displacement or piston meters have undergone various improvements over recent years due to modern advances in materials however, they remain susceptible to mechanical wear and tear. The likelihood that this proven technology will remain in services for many more years, places further emphasis on the need to enhance the related in-service decision-making process.
Limiting the potential for human error adversely affecting the decision-making process can be assisted through the adoption of additional techniques/methods that have a sound theoretical and experimental basis and will complement current practices. These methods and techniques include those related to analysing in-service test results to establish the decay in measurement errors, assessing meter sizing from billing data and understanding the impact that physical water quality has on the accuracy of mechanical meters.
Applying these methods/techniques should facilitate attainment of sustainable benefits for the community and related stakeholders. This is especially relevant as the current national discussion about the cost and reliability of electricity and National Broadband Network (NBN) services has made Australian customers more conscious of their utilities and associated costs. Motivated by the fact that, because of short budgetary cycles, investment requirements in water assets are also not necessarily aligned with long-term customer interests, it has been recommended that pricing should drive efficiency, sustainability and innovation (Infrastructure Australia, 2017).
Achieving a sustainable benefit for the community requires minimising the proportion of customers who will be disadvantaged from potential anomalies in these regulations. This is an alternative approach to that of maintaining the status quo, which generally results in only an average number of customers receiving the benefits of traditionally adopted interventions.
Methodology
The following categories of data were analysed:
In-service accuracy flow tests
Samples of in-service meters were taken from respective cohorts (e.g. populations) for three different Australian meter fleets operating in different climatic regions varying from temperate through to tropical. The Australian Standard AS3565.4 (2007) procedure for testing meters was adopted which involved the following steps:
(Step 1) Grouping of meters according to make, type, diameter, user category, etc.
(Step 2) Determination of a sample size based on their population (e.g. cohort) as specified in the standard.
(Step 3) Selection of meters for removal through the use of a random number generator.
(Step 4) Removal and testing of the sample of meters in a NATA-accredited laboratory at the specified flow rates.
(Step 5) Calculation of the relative weighted errors through the application of the specified default weightings for each flow rate.
(Step 6) Making the decision as to whether the population passes or fails the specified criteria to remain in-service and if the decision is to remain in-service, adopt the additional in-service period or volumetric throughput limit prior to re-testing.
The result of application of this standard is therefore a pass or fail decision in terms of whether the meter population stays in service, or must be replaced with new meters.
This paper describes the further application of the results obtained from these in-service tests.
Meter read data
Quarterly readings for a 3-year period for the respective meter populations were examined. In addition to using this billing data to identify the samples for removal, the average volumetric usage and average volumetric throughput for the population was determined.
Overall approach
The approach adopted involved the following:
- Reference to pertinent aspects of Australian metrological regulations and standards.
- Review of related international research and practices.
- Assessment of meter test results for in-service DN20 mechanical meters representing a total sample size of 1,095 meters from three different fleets. Noting that these tests were undertaken on a NATA-accredited test facility.
- Details of recommended methods/techniques that can complement local regulations and standards when making in-service testing/replacement decisions.
The aim of the approach is to identify techniques/methods that when applied, will complement the current basis that the industry uses for water meter in-service testing and replacement decision making as well as propose a model that quantifies the volume of apparent water losses due to meter errors.
Results
Metrological regulations and guidelines
Meters that are ‘used for trade’ (e.g. custody transfer) are required to comply with the provisions and requirements of the National Measurement Act (1960), as administered by the National Measurement Institute Specifications (NMI-R 49, 2009), in conjunction with Australian Standards AS3565. Exemptions of classes of meters from pattern approval requirements are allowed for in accordance with the National Trade Measurement Regulations 2009.
Guidelines and Codes facilitate consistent approaches for the applicable environment in which they are developed for however, while they are useful, their adoption is voluntary. Water Services Association of Australia (WSAA) Codes of Practice are examples of these non-mandatory guidelines and provide a useful reference.
A meter’s error characteristics are defined according to specific flow rates across the operating range of the meter. The minimum flowrate, designated Q1, is the lowest flowrate at which the water meter is required to operate within the maximum permissible error (e.g.±5%). The permanent or maximum continuous flowrate, Q3, equates to the highest rated operating conditions at which the water meter is required to operate in a satisfactory manner, within the maximum permissible error (e.g. ±2%).The transitional flowrate, Q2, occurs between the permanent flow rate Q3, and the minimum flowrate Q1, that divides the flowrate range into two zones, the “upper zone” and the “lower zone”,
each characterised by its own maximum permissible error
(i.e. ±5% and ±2% respectively).
Relevant aspects of these regulations to this discussion include the following:
- All new cold water meters with a Q3 > 16 kL/h will remain exempt from pattern approved and verification in terms of Australian metrological requirements.
- The population of any new pattern, type or variant of an existing pattern type of meter placed into service shall undergo compliance testing within a period of at least 1 and up to 3 years after being placed into service.
- When the average meter registration for the group of DN20 meters reaches 1,920 kL, or the group age is 8 years, compliance testing is required.
Specific aspects from Australian in-service requirements identified include the following (AS3565.4, 2007):
- Meter in-service testing thresholds for DN20 meters are based on a registered volumetric throughput or equivalent age. Conversion to equivalent age is based on an Australia-wide average annual domestic consumption of 240kL/annum.
- Four-flow rate test points and their associated weightings are specified for accuracy testing.
Measurement error degradation
Weightings are applied to the meter measurement errors established at the various flow rates in a laboratory and used to derive a volumetric error that quantifies apparent water losses (i.e. it converts a meter’s laboratory-determined flow rate errors to an estimated volumetric error). These weightings are defined by the Australian Standards (AS 3565.4) and related guidelines (WSA11, 2012). As mentioned previously in Methodology Step 5, these weightings represent consumption patterns (e.g. water demand profile) that do not necessarily reflect those of all customers within all water utilities in Australia. Applying the four flow-rate test-points requirements of the Australian Standards (AS 3565.4, 2007) and six flow-rate test-point requirements of Australian Guidelines (WSA, 2012) provides an approximation of their underestimation when compared to the nine flow-rate test-point requirement as illustrated in Figure 1. An Australian example of assessing in-service test results for mechanical positive displacement meters identified that the four flow-rate test-point results underestimated weighted relative error by -2.4% when compared to the results of six flow-rate test-point results for the same sample of meters (i.e. for Water Utility A).
Figure 1: Influence number of flow-rate test-points has on weighted relative error (Johnson, 2015)
Water utilities are making decisions regarding the testing and replacement of their in-service (used) mechanical water meters based on compliance criteria and their measurement error decay. A commonly adopted method is to identify a possible relationship between the volumetric throughput or equivalent age of a meter with that of its in-service test results, as determined through the application of AS 3565.4 (2007) and WSA11 (2012).
The evolution of changes in the weighted error with respect to the increase in volumetric throughput or equivalent age of a meter, is known as its error decay or degradation. Whilst testing is undertaken in accordance with Australian standards and guidelines, there is no reference of how to determine the statistical significance of measurement error decay models. This is because the emphasis of AS 3565.4 (2007) and WSA11 (2012) is on whether the population (i.e. meter cohort) passes or fails with respect to remaining in-service.
An example of this is that for a sample size of 229 representing a population of between 10,001 and 35,000 meters (e.g. for Water Utility B), if more than 18 meters fail, then the whole population must be replaced with new meters. This Standard-based decision means that a ‘fail’ result requires that all the meters in the population must be replaced irrespective of whether some might be perceived as being perfectly good. There is a provision in AS3565.4 to redefine the population, or inspect all meters and only remove those that fail, by agreement with the responsible authority (e.g. regulator). However, in this example the utility complied with the outcome of the process, in that the population was deemed to have failed and was removed from the field.
As the meter measurement decay model is based upon a linear regression, testing of the hypotheses about the correlation coefficient is required to measure the goodness of fit of the linear equation assumed for the data (Johnson, 2009). This statistical test is illustrated in Figure 2 and detailed in Appendix A. It is used to test the resultant correlation coefficient versus the sample size as to whether the hypothesis can be accepted or rejected for the selected confidence level. If the correlation coefficient and its respective sample size plot to the right of a confidence level curve in Figure 2, then it is statistically significant at the selected level of confidence.
This validation approach is more objective than guessing whether the correlation coefficient is ‘good enough’ to accept the linear model because of its closeness to unity.
The overall relationship between a selected decay model (in this case, linear), its pre-specified sample size and the correlation coefficient for the specific set of tests is described further in Appendix A.
Figure 2: Linear regression statistical significance
The metrological accuracy requirements of a meter are expressed within an envelope of positive and negative measurement errors; no measuring device can be 100% accurate and record with zero error, even when new. Weighted errors (shown on Y-axis) established for various meters and their respective totaliser throughputs (shown on X-axis) for a sample of meters from a specific population are illustrated in Figure 3. This indicated an average error degradation or decay of -2.7% per 1,000 kL throughput for the sample of meters removed that have previously failed the criteria to remain in-service as specified by the standards. This approximates a measurement error of -0.76% every year for an average consumption of 280 kL/year for mechanical meters.
As the in-service standard’s initial threshold for testing is 1,920 kL, it should be noted that some of these meters exceed this threshold by more than three times. Applying this approach to the data plotted in Figure 2, where 229 meters (n=229) were tested and an R2 of 0.0314 was calculated (via MS Excel) the resultant R value of 0.1772 would be at the 5% level significantly greater than 0 if the sample size was 123 meters. Since 229 meters were tested, the correlation coefficient (R2) of 0.0314 was found to be statistically significant at the 5% level and therefore the error degradation model is statistically significant at the 5% level and can be accepted. The plot is identified in Figure 2 as Water Utility B.
It should also be noted that the 100% errors must be included in the regression analysis as it reflects the failure characteristic of the meter cohort. In terms of the Australian Standard AS3565.4 (2007) it can only be removed as an outlier if the cause has been determined as being unrelated to typical in-service use and not indicative of the general population and in this example there was no justification for the removal of meters with 100% errors.
Application of error decay rates for cohorts of meters to determine the volume of apparent water losses for the whole fleet requires analysis of categories of meters based on their range of volumetric throughput (i.e. totaliser values). The summation of these disaggregated volumetric amounts quantifies the level of apparent losses for the whole fleet.
Figure 3: Example of meter measurement error decay – Water Utility B (Johnson, 2015)
Optimal replacement period
A calculus-derived optimisation model was developed by Noss et al (1987) that utilises an error versus age relationship for meters in service, the rate at which meters fail (stop), together with information on the meter testing, actual costs and water usage, to determine an optimal interval for replacing meters. Inputs required for the model are meter size, type and make of meter. An exogenous variable required is the consumer’s water demand profile (pattern).
A more recent optimisation model that considers the time value of money was developed based on factors that influence the replacement period of water meters, and include the following (Arregui et al, 2010):
- Initial costs covering the acquisition price of a new meter, the installation and administrative costs related to water meter replacement.
- Costs due to unregistered water – considered as a real cost for the water utility. The monetary losses suffered by the utility are proportional to the under-registered volume and the selling price of water.
- Error curve of the meter. Measuring errors depend on the operating flow rate and are defined by the error curve of a meter. The shape of this curve will vary for different working principles and design characteristics of the meters.
- The usage profile of the customer, which statistically is the density function of water use with respect to the usage flow rate.
- The weighted error as a combined error of the meter at different flows considering the percentage of volumetric amount used at each flow rate. Noting that this weighted error includes those that have stopped (i.e. 100% error).
- The water tariff to convert the unregistered water volumes into costs.
- Discount rate or weighted average cost of capital (WACC) to evaluate the future total cost of the unregistered water.
The optimal meter replacement period can be established from the net present value of the meter replacement chain (NPVCn) defined by the following formula developed by Arregui et al (2010).
NPVCn
Where:
NPVCn = present value of the costs of infinite replacements conducted at fixed intervals of time ($)
Cacq= Cost of meter acquisition (e.g. purchase) in $
Cinst = Cost of meter installation in $
i = average volume of water used in a year i (kL)
Ɛi = weighted error for the meter in year i (%)
Cw = average price of water ($/kL)
r’= real discount rate (e.g. WACC) (%)
n= number of years of the meter replacement period
An example of the optimal replacement period analysis for a cohort of mechanical meters that have a decay rate of -2.7% per 1,000 kL throughput is illustrated in Figure 4.
Figure 4: Example of a theoretical optimal replacement period – Water Utility B
Where the hypothesis is rejected for the application of a linear regression model and hence cannot be applied to establish the error decay, optimisation theory can also be used to approximate the error decay from an adopted replacement period. This was the case for Water Utility A shown in Figure 2, which had a pre-defined testing trigger of 18 years. As the linear regression model could be adopted for utilities B and C as illustrated in Figure 2, the optimal replacement periods could be established for the respective error decays as given in Table 1.
Physical water quality
A comparison of physical water quality characteristics, together with the respective error model and average throughput for three water utilities, is given in Table 1. It would appear that higher free chlorine concentrations and water temperatures have an influence on the error decay model through accelerated wear and tear of positive displacement mechanical meters.
Table 1: Physical water quality and error decay results comparison
Assessments based on billing data
Meters that are found to be too large (e.g. oversized) for measuring the diurnal water usage pattern of a particular customer will generally result in larger volumes of non-registered water. This is because the meter cannot monitor the flow gap between zero flow and when the mechanism commences operating and starts recording usage. These over-sized meters also tend to have an increased measurement error of the volume of water measured at low flows (e.g. increased under-registration).
Undersized meters that operate at or above their maximum or overload flow rate (e.g. Q4) tend to suffer excessive mechanical wear and tear with an increased rate of measurement error decay. The metrological description for overload flowrate is the highest flow rate, at which a water meter is required to operate, for a short period of time, within its maximum permissible error. Although there is some debate as what this “short period of time is”, some meter manufacturers are of the opinion it is a total of 24 hours in the design life of a meter. Meters that are identified to be continuously operating near or at Q4 should be upsized or replaced with a meter of the same size but with a larger flow range capability (e.g. R ratio).
Generally, a billing system is the only source of readily available historic data for the whole meter fleet. Analysis of this data can facilitate strategic decisions regarding the correct sizing of meters and forecasting meter testing triggers or thresholds.
A commonly adopted approach to assessing whether the flow range capability of a cohort of in-service meters match customer’s usage patterns is the analysis of the accumulated registered volume and the age of the meter so as to determine whether it is subject to higher or lower flowrates than the recommended ones (Arregui,et al, 2006). Madrid’s water authority adopted a similar approach that examined bimester readings (i.e. two readings a year) (Canal de Isabel II, 2010). These references were assessed to establish an approximation of the range within which in-service meters should be operating when examining their existing throughputs. This is detailed in Table 2. The data for DN20 to DN40 sizes relates to single jet meters and the DN50 meter relates to Woltmann turbine meters. Arregui et al (2006) does not specify the billing period for the meter reading.
These findings from international investigations can be applied as approximate thresholds or benchmarks for sizing in-service meters. If the average flow rate, derived from billing data for a low usage season (e.g. billing period), was less than the lowest benchmark (i.e. approximately <1.0%) and for a high usage season, greater than the largest value (i.e. approximately >10%) for DN20 meters, then the current meters are likely to have an inadequate operating range to match their historic range of water usage. This analysis does not necessarily provide a precise answer for an individual meter however, can be used for a direct follow-up analysis of a specific customer’s billing records or field logging exercises to correctly size the meter.
Analysis of billing data from a sample of Australian utilities from DN20 positive-displacement mechanical meters, found that generally the lower benchmark was met (i.e. approximately 1%) however, the higher benchmark was found to be considerably larger (viz. >40%). This could be the result of higher water usages in Australia compared to that of Europe and/or different meter reading (e.g. quarterly) periods. However, considering a selected sample of Australian in-service mechanical meters they would appear to be operating at or over their metrological flow range capabilities resulting in an increased rate of mechanical wear and tear.
Table 2: Meter operating range based on billed usage
Billing data assists in categorising meters according to volumetric throughput (i.e. totaliser) ranges. The summation of volumetric amounts determined from the error decay relationship quantifies the level of apparent losses for the whole fleet.
Non-mechanical meters
Non-mechanical meters such as those operating with solid-state digital electronics that have no moving parts (e.g. static meters) are generally not subject to the same measure error decay characteristics as those of mechanical meters. However, these electronic meters can be subject to the following:
- A sudden ad hoc change in the meter accuracy resulting in a shift in bias (systematic) errors that is not always immediately apparent to the operator/customer. Some digital/electronic meters have an in-built self-checking capability that prevents bias (systematic) measurement errors and this capability is disclosed by the particular meter manufacturer.
- Sampling frequency of the ultrasonic or electromagnetic ‘beams’ measuring the flowing water. The greater the frequency of scans to measure flow rates and the resultant volumetric amount, the less variability in the results and the greater the accuracy. Scanning frequency also relates to power requirements, as generally the greater the scanning frequency, the greater the power requirements for some types of meters.
As a new pattern or type of meter, or as a variant of an existing pattern type, non-mechanical meters might still be required to undergo compliance testing within a period of 1 to 3 years of being placed into service.
Discussion
Australian standards and guidelines were developed based on data derived from using and testing mechanical meters over many years. Time periods, test flow-rates, weightings and decision rules are intended to apply to mechanical meters based on reasonably well known and predictable failure modes and performance deterioration. As such, these aspects of the standard may not be appropriate for statistically monitoring the performance of a population of non‐mechanical meters. However, some of the generalised default values used for average usages and demand pattern weightings might also not be appropriate for application for in-service decision making for all mechanical meter applications.
All new cold water meters with a Q3 > 16 kL/h are exempt from pattern approved and verification in terms of Australian metrological requirements which includes exemption from in-service testing requirements. Unless specified at procurement, this means that all meters larger than approximately DN40 are not required to undergo in-service testing, which introduces potential risks for the utility and its customers.
In-service testing requirements based on the four-flow rate point tests underestimate the measurement error when compared to the six-flow rate point test results. This is because the six-flow tests cover a greater range of the meter’s operation and reflect a better representation of in-service flow conditions.
Testing the statistical significance of error decay models can supplement the guidance provided by current in-service standards in making meter testing and replacement decisions. Assessing the statistical significance of a linear model is based on sound statistical theory and provides a more objective assessment as to whether or not a model should be adopted.
Application of optimal replacement theory that considers the time value of money can also assist in providing a perspective on previously adopted replacement periods as well as identifying the impact that error decay rates have on meter replacement periods.
There is some indication that relatively high water temperatures and free chlorine levels could have an adverse influence on the measurement error decay rates of positive displacement mechanical meters. A subjective assessment of the physical water quality characteristics and the error decay rates is that there could be a marked acceleration in mechanical wear and tear for mean water temperatures greater than 25ºC and free chlorine levels above 1 mg/L.
Analysis of readily available billing data can provide an important source of information that can aid decision making regarding the matching of meter flow range capabilities with respect to a customer’s historic water usage records. Comparisons of the analysis of billing data with internationally established thresholds does not necessarily provide a precise answer for an individual meter. However, it can be used to direct follow-up analysis of a specific customer’s billing records or field logging exercises to correctly size the meter.
Conclusion
Methods and techniques identified that could complement the decision-making process related to current meter in-service testing and replacement requirements include the statistical analysis of test results, assessment of meter sizing from billing data, application of optimisation theory and understanding the impact physical water quality has on the accuracy of mechanical meters. In particular:
- Testing the statistical significance of error decay models based on fitting a linear regression curve to the results of a six-flow rate point test provides a more accurate and objective approach to assessing the relative weighted error and the volume of apparent water losses.
- Optimal replacement theory that considers the time value of money provides a useful technique to identify the impact that error decay rates have on meter replacement periods.
- There is an indication that physical water quality characteristics such as high water temperatures and high levels of free chlorine accelerate wear and tear of mechanical meters.
- Comparison of a meter’s flow range capabilities with that of a customer’s historic water billing records facilitates identifying those cohorts potentially subjected to an increased rate of mechanical wear and tear.
Applying these methods/techniques facilitates optimal decision making regarding the in-service testing and replacement decisions, thereby ensuring that all metered customers and related stakeholders receive sustained additional benefits due to improvements in meter accuracies.
Acknowledgements
Associate Professor in Statistics, Dr Andrew Metcalfe, School of Mathematical Sciences, the University of Adelaide is gratefully acknowledged for his review of the statistical significance of the linear model detailed in this paper. The review and proof reading of this paper by Keith Roberts and Greg Lutton is also gratefully acknowledged.
About the author
Edgar H Johnson | Edgar is a professional engineer with more than 35 years of Australian and international experience in water management. His education combines a Doctor of Technology with a commerce degree, providing him with unique insight into the full range of utility system practices. He has developed standards and guidelines associated with water loss management, efficiency and metering and has published more than 30 related articles/papers/research books. His involvement with the International Water Association (IWA) Water Loss Specialist Group included leadership of its non-revenue water apparent loss (AL) initiative. He was selected as one of 30 of Australia’s Most Innovative Engineers by Engineers Australia in 2017. Edgar is currently a Senior Technical Director: Water Efficiency at GHD.
References
Arregui F., Cabrera E. & Cobacho R.(2006). Integrated Water Meter Management. IWA Publishing. (ISBN:1843390345)Arregui F., Cobacho R., Soriano J. & García-Serra J.(2010) Calculating the optimum level of apparent losses due to water meter inaccuracies. IWA Water Loss 2010 Conference. Manila.
Australian Standard AS 3565 – Meters for cold and heated drinking and non-drinking water supplies.
- Part 1 (2010): Technical Requirements
- Part 4 (2007): In-service compliance testing
Infrastructure Australia (2017). Reforming Urban Water – A national pathway for change. Australian Government.
Johnson, E.H. (2009). Management of Non-revenue and Revenue Water Data. Engineers Media, Australia. ISBN 9780858258839 (2nd Edition).
Johnson, E.H. (2015). Innovative Meter Fleet Condition Assessment Identifies Non-revenue Water Volumes. IWA Efficient 2015 Specialist IWA International Conference. Cincinnati, USA.
NIV Bible (1984). The Holy Bible, New International Version. The Bible Society in Australia. Proverbs 11:1, Proverbs 16:11; Proverbs 20:10.
Noss R.R., Newman G.J. & Male J.W. (1987). Optimal Testing Frequency for Domestic Water Meters. Journal of Water Resources Planning and Management Vol 113, No. 1 January.
WSA 11 (2012). Compliance Testing of In-Service Water Meters – Code of Practice. Water Services Association of Australia.