Cost-Effective Chlorination Strategies for Drinking Water
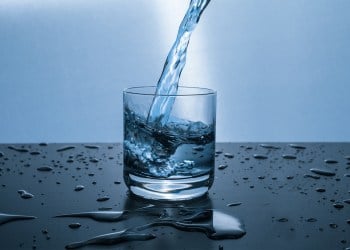
Meeting chlorination disinfection goals in drinking water distribution systems.
DOWNLOAD THE PAPER
Abstract
After outlining three major goals for secondary disinfection, this paper describes a procedure to search for and identify cost-effective chlorination strategies to meet these goals within multiple-source distribution systems. This procedure is based on new, accurate and efficient process models of chlorine decay and disinfection by-product formation, which have been shown to apply over the full range of operating conditions.
To gain the full benefits of the procedure, these new models need to be embedded in the Multi-Species eXtension (MSX) within the EPANET distribution system simulation package, or one of its commercial derivatives. (The authors have tested and applied them within the Innovyze H2OMAP MSX environment).
If no chlorination strategy can be found that meets the defined disinfection goals at the current level of treatment, one option is to improve the treatment to produce more chlorine stable water. Models to predict the degree of removal were combined with the chlorine-decay and DBP-formation models to form an augmented procedure that accounts for the effect of additional removal.
The other major option is to replace chlorination with chloramination, for which adequate process models are still under development. Applications of these procedures to real Australian distribution systems are also summarised.
Introduction
Chlorination is the cheapest and generally most effective method of achieving primary disinfection of drinking water, and to inactivate the pathogenic microorganisms that remain after treatment. It also has the advantage of providing a persistent residual in the water as it travels through the distribution system to every consumer to inhibit the regrowth of microorganisms in bulk water and on pipe walls (secondary disinfection).
However, free chlorine reacts with many other chemical substances left in the water after treatment, leading eventually to loss of its concentration and power of disinfection. Consequently, the major goal for secondary disinfection is to maintain more than a minimum (free) chlorine concentration throughout the distribution system. This is likely to remain so for distribution systems with longer retention times or in low- to mid-latitude locations with higher water temperatures.
In recent decades, formation of some disinfection by-products (DBPs) of chlorination – such as trihalomethanes (THMs) – has become recognised as harmful to human health. Limits have therefore been set on their concentrations by regulators such as the EPA in the US and Departments of Health in Australia through the Australian Drinking Water Guidelines (ADWG). Consequently, a second health-related goal for secondary disinfection is to keep DBP concentrations below regulated limits. This goal is more easily met in Australia because the AWDG guideline for THMs (0.25mg/L) is much higher than the limits set in many other countries (0.1 mg/L or less).
The Australian Guidelines also include an aesthetic limit on chlorine concentrations to recognise that consumers are sensitive to relatively low chlorine concentrations. Consequently, a third goal for disinfection is often to keep chlorine concentration below taste/odour thresholds. This and the second goal are in conflict with the major goal of maintaining a minimum residual.
A chlorination strategy is a pattern of dosing (comprised of selected locations and doses) imposed within a distribution system. A cost-effective chlorination strategy is one that minimises the cost of achieving the disinfection goals for a given flow/temperature scenario. Different scenarios will therefore have different cost-effective dosing strategies. If the introduction of new booster stations is to be considered when developing these strategies, then the associated capital costs need to be included in the evaluation, in addition to the operating costs of all stations involved.
Accurate numerical models of chlorine and DBP concentrations are essential tools for developing chlorination strategies so that they can be shown to meet the disinfection goals and their costs can be calculated. Sufficiently accurate and computationally efficient models are required so that numerous alternative strategies can be developed and compared to find the one that minimises the costs for each flow/temperature scenario.
Robust hydraulic models of distribution systems have been available for more than 25 years. These models predict the flows and pressures that result from a time-varying set of downstream demands. In a “system modelling” software package, these hydraulic models are accompanied by transport/mixing models, which route a dissolved inert substance through the network of pipes and tanks, and process models, which account for the gain or loss of a reactive substance such as chlorine within each routed bulk-water “parcel”. Additionally, interaction between water parcels and the pipe-wall (“wall reaction”) may be included. EPANET (Rossman, 1994) is probably the best-known and most widely used of these system modelling packages, partly because there are many other more user-friendly commercial packages based on this public-domain software.
Simplistic process models of chlorine decay were made available in the first version of EPANET (Rossman, 1994) and a DBP formation model was proposed in EPANET 2 (Rossman, 2000). However, in addition to their inaccuracy and inefficiency, these are limited to being functions of the concentration of only a single species (e.g. chlorine or DBPs). Consequently, it is not possible to conduct the simultaneous modelling of chlorine and DBP concentrations required for strategy development in EPANET, EPANET 2 or their direct commercial derivatives. Similarly, it has not been possible to model chlorine (or DBP) concentrations in systems supplied with water from multiple sources of different qualities.
New bulk-water decay models with accuracy and efficiency sufficient for strategy development have only been rigorously established within the last few years (Fisher et al. 2016). More recently, Fisher et al. (2017) presented a validated, robust model of chlorine decay due to interaction with lined pipe-walls (termed EXPBIO). This model contradicts both of the alternative non-validated models available in EPANET, which are currently in worldwide use. A similarly validated model for unlined iron pipes is still not available.
We first outline these new, accurate, efficient models of chlorine decay in bulk-water and lined pipes. The simplest realistic model of trihalomethane formation that can be used in association with these chlorine-decay models is also presented. We then consider how these models can be simultaneously implemented within the Multi-Species eXtension (MSX) to EPANET 2 (Shang et al. 2008) or its commercial derivatives, so that dosing strategies can be rigorously evaluated and the cost-effective ones can be identified. In addition, we show that the same approach can be used to simulate chlorine residuals and THM formation in multiple-source distribution systems.
If no dosing strategy can be found that meets the disinfection goals simultaneously, one possible solution is additional removal of reactants such as DOC (Kastl et al., 2004), or their pre-oxidation (Kastl et al., 2015), to lower the chlorine decay rate. We also show that coupling models of reactant removal with the new model of bulk decay allows prediction of the resulting improvement in chlorine residuals and THM concentrations in the distribution system. The problem of optimising strategies for the other major alternative of chloramination is also briefly considered.
Procedure to model chlorine decay
We must first distinguish between “bulk decay” – reactions of chlorine with substances left in water after treatment (termed “reactants”) – and “wall decay” – reactions with pipe-wall surfaces and substances adhering to them. Total decay of chlorine is the sum of these two components (Eq 1, Table 1). Figure 1 illustrates the relationship between these variables and their measurement.
Figure 1. Relationship between bulk and wall decay models and data for their derivation
Conduct decay tests on bulk water
Bulk decay is first measured in decay-tests on samples of water taken immediately after treatment, but before chlorination (Figure 1). In a laboratory, their sub-samples are subjected to different combinations of temperature, initial chlorine concentration and booster concentration(s), and the subsequent chlorine concentration is monitored over time until each sub-sample decay rate becomes negligible.
Fit bulk-decay model to decay-test data
The augmented two-reactant (2RA) model is the only available accurate, efficient, rigorously validated model of chlorine decay in bulk water (Fisher et al. 2016). By “rigorous validation”, we mean that the predictions from a fitted decay model are compared with decay tests not used in the fitting procedure, for a range of different waters.
The two-reactant (2R) model assumes that chlorine reacts with two groups of compounds – fast-and slow-reacting – at rates defined by classical chemical theory (Eqs 3 and 4, Table 1). The augmented (2RA) model allows the two decay-rate coefficients to vary with temperature according to the classical Arrhenius relationship (Eq 5, Table 1).
By “efficient”, we mean that the bulk-decay rate in a single source water can be accurately represented by a single set of values of the five parameters involved, over the entire operating range of time, temperature and initial and (multiple) booster doses (Fisher et al., 2016). Figure 2 illustrates the accuracy of 2RA model estimation/prediction for combinations of these variables, using a single set of parameter values. In addition, chlorine decay in blends of pairs of waters of any proportion is accurately represented by volumetric averaging of the concentrations obtained from coupled 2RA models of the individual components (Fisher et al. 2015). This is a critical prerequisite for accurate representation of bulk decay in systems with parallel paths/loops or multiple sources.
Figure 2. Chlorine concentrations from 2RA model, calibrated against the Greenvale data set comprising decay tests from (A) five initial doses (IDs) at 20ºC plus two boosted and (B) two IDs at 15ºC and 25ºC plus one boosted. Colours: rechlorination – green, ID temperature – red to blue; dash length – ICC magnitude. Legend – ICC, water temperature and rechlorination time (hours after ID).
By “fitting” a decay model, we mean finding the values of the five parameters that best describe bulk decay-test data from a single source-water. This involves systematic selection of trial parameter values and minimisation of the sum of differences between the resulting decay model predictions and decay-test data. The fitting procedure requires a minimum of four decay tests, conducted using each combination of two (constant) temperatures and two initial chlorine concentrations (ICCs). If rechlorination is to be considered, at least the high-ICC, high-temperature decay test should be continued after substantial decay of the initial dose (Fisher et al. 2016).
Methods for solving decay model equations over time
There is no analytical solution available for the 2R or 2RA models (Eqs 2-5, Table 1). However, there is an approximate solution for the 2R model, which generally provides good estimates of the parameters using the Solver routine within an Excel spreadsheet (Kopaei and Sathasivan 2011). Alternatively, any software that can iteratively minimise the differences between 2RA model solutions and decay-test data can be used for this purpose. In our experience, the AQUASIM software (Reichert 1998), now freely downloadable, is a general, robust and convenient environment for carrying out this parameter optimisation, especially when rechlorination data is involved.
Set up and run the bulk-decay only model
The bulk-decay only model can be set up and run within the MSX module of EPANET 2 or one of its commercial derivatives (Innovyze InfoWater MSX or Bentley WaterGEMS MSX). Fisher and Kastl (2013) presented a detailed procedure for setting up the 2RA bulk-decay model in Innovyze InfoWater MSX. This includes specification of the process equations and their parameter values, as well as types of dosing inputs.
Conduct field measurements at key system locations
Wall decay can only be calculated as the difference between the total decay measured between two points in a distribution system and the modelled bulk decay between the same points (Figure 1). Consequently, there must first be in-system measurements of chlorine concentration available from several key locations, which provide increasing water age in a known flow regime, to enable fitting (calibration) of any wall decay model.
Identify “key sites”
Identify the locations where the bulk-decay model predictions first diverge from the in-system measurements. Then identify locations further downstream of each of these “divergent” locations where free chlorine first becomes negligible (say <0.1mg/L). Figure 3 illustrates the variation of wall-decay rate (rw) measured along a series of lined pipes (assuming a different wall decay rate kw0 in each) compared with the new EXPBIO model predictions (Fisher et al. 2017). As this implies chlorine concentration decreases approximately sigmoidally with increasing water age, the key measurement sites for fitting the EXPBIO model would then be mid-way between pairs of corresponding “divergent” and “negligible” chlorine sites. These “key sites” are expected to be close to where the maximum specific wall-decay rate [mg/dm2/h] would occur.
Figure 3. Quantified wall-reaction rates (kw0) along Mirrabooka pipe run compared with EXPBIO model predictions (rw). Source: Fisher et al. (2017)
Set up and fit the EXPBIO model to the in-system chlorine measurements
The EXPBIO model (Fisher et al. 2017) has three parameters (Eq 6, Table 1). One of these is the mass transfer coefficient (km), which is available as a variable from within the EPANET hydraulic model, so does not have to be fitted. The other two parameters (A and B, Eq 6, Table 1) are fitted by minimising the errors between the in-system measurements of free chlorine concentration and those obtained from numerical solutions of the combined “bulk (2RA) plus wall decay (EXPBIO)” models at the same measurement sites. Particular attention should be given to predicting the data from “key sites” so that accurate estimates of near-peak wall-decay rates are included in these solutions.
Validate “bulk plus wall” decay models
Bulk plus wall decay models should be validated against an independent set of in-system measurements under another flow/temperature scenario. This validation is particularly to determine whether the EXPBIO wall-decay model parameter values are reasonably stable or need to be re-calibrated under different scenarios.
The MSX system models are now ready for cost-effective chlorination strategy development with the goals of maintaining a minimum target residual throughout a single- or multiple-source system, while not exceeding an aesthetic limit immediately downstream of any chlorine dosing points. The EPANET MSX User Manual (Shang et al. 2011) gives details for setting up different types of mass injection of chlorine at any chosen booster sites.
Procedure to model trihalomethanes (THMs)
Clark (1998) proposed that THM formation in a given water could be predicted as a constant yield (mg THM/mg Cl consumed) from chlorine decay reactions. Boccelli et al. (2003) confirmed this relationship in several US waters, but also showed that the yield differed between waters. This principle is applied with the 2R(A) model to produce the THM formation model of Eq 7, Table 1.
The yield for a given water is estimated by taking subsamples for THM analysis at chlorine sampling times near the start and end of a decay test. A third such sampling at an intermediate time is often done to check for linearity over the entire range of chlorine consumed.
Set up DBP formation model in MSX
In earlier studies and consulting projects, we have assumed that both fast and slow bulk reactions generate THMs. Whether the same yield applies to both is still unknown. Similarly, whether a different yield is obtained from wall reactions remains to be shown – few THMs should be generated in unlined iron pipes.
A similar approach can be taken to modelling haloacetic acids (HAAs), the other major regulated group of DBPs. However, in addition to the uncertainties just mentioned, there is some evidence that HAAs are degraded by biofilms on pipe-walls, so that a more complex formation model would be required. This is not a priority issue in practice,
as the THM limit is far more often exceeded than the HAA limit is.
Chlorination strategy optimisation
The validated MSX system models are ready for development of cost-effective chlorination strategies that simultaneously achieve all three disinfection goals under nominated flow/temperature scenarios in single- or multiple-source systems at minimum cost.
The simplest optimisation procedure involves the following steps:
- Set up and calibrate the hydraulic model of the system within EPANET or one of its commercial derivatives that also contains MSX.
- Define a flow/temperature scenario that is thought to be the “worst-case” for chlorine decay, e.g. late summer, when demands are lower than the maximum, but temperatures are still high. Ensure that realistic flows are obtained from hydraulic model simulations by comparison of model predictions with flow/pressure data.
- Set up and determine parameters for chlorine-decay and DBP-formation models in MSX, following procedures described above.
- Ensure that realistic chlorine and DBP concentrations are obtained from respective model simulations by comparison of model predictions with online and/or grab-sample data. This validation would require using current chlorine doses as model inputs (“base case”).
- Set up an operating cost model for existing chlorination plants (at WTPs and downstream booster locations).
- Generate operating costs for base case (over daily/weekly hydraulic cycle). Assume sunk capital costs, i.e. existing capital costs, will be included in all dosing strategies.
- If disinfection goals are not met by the existing dosing strategy, modify the strategy by increasing doses at WTPs/boosters and/or introducing new boosters until water quality simulation shows that disinfection goals are met. Reductions in retention time (in service reservoirs) should be considered as part of these modifications. Generate capital and operating costs for this feasible strategy.
- Repeat step 7 to reduce capital and operating costs as far as possible, which results in the most cost-effective strategy for this scenario.
- Synthesise a “best-case” scenario, in which chlorine decay is likely to be least, e.g. winter temperatures, but still moderate flows.
- Repeat steps 7 and 8, including only sub-sets of booster locations required by the worst-case cost-effective strategy. This results in the best-case cost-effective strategy.
The cost-effective strategies for these two scenarios define the extremes between which disinfection goals would be met cost-effectively. There are other intermediate scenarios for which different strategies will be cost-effective, which can be found by repeating steps 9 and 10. A long-term amortised cost analysis, to minimise combined capital and operating costs, would require inclusion of an ensemble of such strategies that is likely to be used over a decade or more.
When deriving a cost-effective strategy for a given scenario (Step 7), several explicit optimisation techniques have been proposed for locating and scheduling booster dosing plants. For example, Devi Prasad et al. (2004) minimised total mass injection rate of chlorine within constraints on both chlorine residuals and THM concentrations. However, almost all of these assume first-order chlorine decay kinetics and linear superposition of the effects of any number of booster plants at any node downstream of them, which gives unrealistic results for both chlorine concentrations and THM formation. Furthermore, they do not account for fixed operating costs (such as maintenance visits) or any capital costs (such as booster equipment, buildings or land). Consequently, applications of such techniques should be regarded with considerable caution.
No feasible chlorination strategy?
It is quite possible that no chlorination strategy can be found which satisfies the disinfection goals simultaneously because there is too much dissolved reactant left in the water after treatment.
One possible solution is to remove or pre-react more of these (predominantly organic) reactive substances at the WTP before primary chlorination. Enhanced coagulation, in which coagulant above that required for colour/turbidity removal is added at controlled low pH, is probably the cheapest and simplest alternative for a conventional WTP. It is also recommended by the USEPA as best available technology.
Kastl et al. (2004) developed a model that predicts the degree of removal of dissolved organic carbon (DOC) that can be achieved over the range of practical combinations of alum or ferric coagulant dose and pH. As this potential DOC removal is dependent on the nature of the DOC, the parameter values for a particular source water must be determined from a handful of jar tests. Figure 4 illustrates the results obtained from this model using alum as a coagulant. Although this example is for a relatively high level of initial DOC, Kastl et al. (2004) showed the removal model to be accurate over (at least) the range of 2.3-12.7 mg/L initial DOC. Models of other DOC removal processes could be developed if such processes were under consideration for other reasons.
Figure 4. Modelled dissolved organic carbon (DOC) removal by enhanced coagulation with alum at different pHs. Source: Fisher et al. (2004)
The effect of the degree of DOC removal on chlorine decay on the parameters of the 2RA chlorine-decay model can be determined from a new set of decay tests conducted on the water produced in the previous jar tests (Fisher et al. 2004). Then steps 7-10 in the strategy optimisation procedure above can be repeated using the new 2RA model parameters to find cost-effective strategies that are now feasible due to the additional DOC removal.
If no feasible strategy can be found after the effect of enhanced coagulation is included in the modelling/ optimisation procedure, then the same approach to incorporating the effect of additional removal processes such as ozonation and/or activated carbon adsorption can be taken.
The other major alternative to additional treatment is to adopt chloramination, instead of chlorination, as the secondary disinfection process. While chemical decay of chloramine is much slower, the process of maintaining a minimum residual is more complex and costlier to manage than chlorination is. In addition, chloramine is susceptible to microbial decay (Sathasivan et al., 2005), which can be more than an order of magnitude greater than the chemical decay. The microbiological processes involved are not well enough understood to include in process models within system simulation software, although substantial progress in this direction has been made over the last decade (e.g. Sathasivan and Bal Krishna 2013). These developments are sufficiently a complex and different from the chlorination case that they require separate presentation in future. However, an optimisation procedure for chloramination, analogous to that described for chlorination, is not fully feasible at this time.
Application of the optimisation procedure in real systems
This optimisation procedure has been developed and applied successfully to real distribution systems for more than twenty years. Projects conducted on some of these systems are briefly characterised in Table 2 to illustrate the range of issues that have been successfully addressed with these procedures. These range from modelling chlorine concentrations alone in a simple system (in the development of the new wall-decay model) to calibrating and validating process models of bulk and wall decay along with trihalomethane formation, in a system with multiple ground- and surface-water sources of distinctly different quality. In some cases, the effects of additional treatment on in-system chlorine concentrations were to be determined before the treatment was carried out. In another, the decision to convert a chloraminated system back to chlorination was based on whether predicted minimum in-system concentrations could be achieved with chlorination of the water source instead (Fisher et al. 2009).
Conclusion
A cost-effective chlorination strategy is defined as one that minimises the cost of achieving the disinfection goals for a given flow/temperature scenario within a distribution system. After outlining three major goals for secondary disinfection, a procedure is described to search for and identify cost-effective chlorination strategies within multiple-source distribution systems.
This procedure, based on new, accurate and efficient process models of chlorine decay and disinfection by-product formation, has been shown to apply over the full range of operating conditions. To gain the full benefits of the procedure, these new models have been embedded in the Multi-Species eXtension (MSX) within the EPANET distribution system simulation package, or one of its commercial derivatives.
If no chlorination strategy can be found that meets the defined disinfection goals, at the current level of treatment, one option is to remove models to predict the removal of the dissolved reactants remaining after treatment. Models to predict the degree of removal were linked with the chlorine-decay and DBP-formation models to form an augmented procedure to account for the effect of additional removal in the optimisation procedure (Fisher et al. 2004).
The other major option is to replace chlorination with chloramination. However, chloramine decays due to both chemical and microbial processes. Microbial decay can easily be more than an order of magnitude greater than chemical decay. Using the same approach to finding cost-effective chloramination strategies is not fully feasible at present, due to inadequate understanding of the microbial processes involved in chloramine decay.
The procedures described have been applied to a wide range of chlorination issues in real Australian distribution systems, with considerable benefits accruing to the system owners. There are many other systems worldwide that could similarly benefit from such applications.
Acknowledgements
The accurate, efficient models described in this paper had their origins within the Research Program of the Cooperative Research Centre for Water Quality and Treatment. The data for their validation was also collected within this Program, with considerable in-kind contributions from Sydney Water, Melbourne Water, WA Water Corporation and SA Water. The authors were responsible for the later validation and publication of the models with permission from the relevant water utilities. Applications of the new process models within AQUASIM or H2OMAP-MSX models of the systems listed in Table 2 have been conducted within consultancies for water utilities as clients. Lead consultants for each application are acknowledged in the Table 2 Notes.
About the authors
Ian Fisher | Ian graduated in Engineering from Melbourne University and obtained a PhD in Water Engineering from the University of NSW, Australia. As Principal Scientist/Consultant with Sydney Water Corporation for almost twenty years, he led major projects in measurement and modelling of water quality in lakes, reservoirs, rivers, estuaries and distribution systems. In addition, Dr Fisher was the Foundation Coordinator of the Distribution System Research Program in the national Cooperative Research Centre for Water Quality and Treatment (CRCWQT). The innovative chlorine-reaction,
by product-formation and carbon-removal models described in this and a series of earlier papers were developed largely within the CRCWQT Research Program. Dr Fisher continues his work with colleagues to apply these models, and techniques assessing chloramine decay, through his consulting company Watervale Systems and in co-operation with Western Sydney University.
George Kastl | George is a chemical engineer with over thirty years’ experience, mainly in the water industry with Sydney Water, WorleyParsons and MWH. He is currently a Research Fellow in the School of Computing, Engineering and Mathematics, Western Sydney University. His recently completed PhD studies at the University of Western Sydney focus on organic carbon removal during water treatment.
Arumugam Sathasivan | Arumugam is a Professor in the School of Computing, Engineering and Mathematics, Western Sydney University. He is continuing the research carried out within the CRCWQT to stabilize disinfectants (chlorine and chloramine) while minimising the negative effects (DBP formation, bacterial regrowth), through more fundamental understanding of treatment and distribution system processes and development/application of process models.
References
-
Original second-order bilinear bulk decay model included two organic nitrogen reactants as well as two organic carbon reactants.
-
The system network flows and quality were represented within the AQUASIM software package (Reichert 1998). We also use another module of AQUASIM for estimation of parameter values of all bulk-decay models from decay-test data.
-
The “equivalent diameter” (Deq) model was an early representation of wall decay which simply accelerated (2-reactant) bulk decay. It is superseded by the EXPBIO model of Fisher et al. (2017).
-
DSMtool is a system simulation package which replaced the decay models within EPANET with the 2-reactant and Deq decay models. It is superseded by the MSX package (Shang et al. 2008).
-
mEnCo is the software package that determines the degree of removal of organic carbon due to enhanced coagulation with alum or ferric compounds at controlled low pH.
-
H2OMAP-MSX is a commercial version of EPANET-MSX, now available as Innovyze’s InfoWater MSX, which includes GIS representation and other user-friendly features.
-
Lead consultant: MWH Global
-
Lead consultant: WorleyParsons
-
Lead consultant: Engeny Water Management